Uncovering AI Bias: Unraveling the Invisible Culprit
- Published June 7, 2024
Uncovering AI Bias: Unraveling the Invisible Culprit
In our ever-evolving world, increasingly artificial forms of intelligence are tasked with making complex decisions. As various literature indicates Artificial Intelligence (AI) is now being used in various domains such as Business, high-stake decision-making, and over the past few years in the medical sector.
With this growing prevalence, however, people have noticed that these systems have shown signs of prejudice, through observed sexist and discriminatory behaviour. The recent European AI Act, also covers the matter of such prejudice rather extensively and sets a foundation for tackling problems associated with it.
Technical documentation tends to use the term “bias” to describe this skewed type of behaviour towards certain demographics; However, as it stands, there are varying definitions of bias which can be the cause of much confusion.
This article aims to provide a clear, concise understanding of bias in AI. We’ll introduce methods to measure and minimize bias and explore the role of synthetic data in this path to fairer systems. Additionally, we’ll highlight how Syntho, can contribute to this effort. So, whether you’re a practitioner looking for actionable insights or just curious about this topic, you’re in the right place.
Table of Contents
Bias in Action: A Real-World Example
Why should anyone care about biased behavior in AI? Our world may be inherently biased, but the impact of AI bias is far-reaching and often invisible yet potent. Bias in AI is not just an academic concept; it’s a real-world problem with serious consequences.
Take the Dutch child welfare scandal as an example. An automated system designed to generate fair and efficient results wrongly flagged thousands of parents for fraud due to flawed data and assumptions. The result was families thrown into turmoil, damaged personal reputations, and financial hardship—all due to biases in an AI system. This example underscores the urgency of addressing bias in AI.
The above mentioned is only one example; AI bias impacts many areas of life, from hiring decisions and loan approvals to medical treatment. Biased AI systems can perpetuate existing inequalities and create new ones.
Specific types of biases, such as historical bias and measurement bias, lead to flawed decisions. These biases are inherent in the data, rooted in societal biases, and reflected in unequal outcomes among different demographic groups. They can skew predictive model decisions and result in unfair treatment. Although this post does not cover specific biases in detail, additional resources are provided for those interested in exploring the field further.
In the grand scheme, AI and bias can act as a silent influencer, subtly shaping society and our lives, often in ways we don’t even realize. This might lead you to question why no action has been taken to stop it and whether it’s even possible to do so. With technological advancements, tackling this problem is becoming more feasible. However, the first step is to understand and acknowledge the existence and impact of AI bias.
Definition and types of bias in AI
- Data to Algorithm: These biases originate from the data itself, whether due to poor data collection or inherent biases in the world.
- Algorithm to User: These biases stem from the design and functionality of the algorithms. They include how algorithms interpret, weigh, or consider certain data points over others, leading to biased outcomes.
- User to Data: These biases arise from user interactions with the system. Users’ data input methods, inherent biases, and trust in system outputs can influence outcomes.
Measure Twice, Cut Once
Now, with our formal definition of bias, let’s establish a framework to identify the extent to which bias is present in our systems. When correcting for biases in machine learning models, our goal is to maintain predictive performance while increasing fairness. To achieve this, we need performance metrics to evaluate model effectiveness and fairness indicators to assess bias.
Thus, we have two components: performance metrics and fairness metrics. Various studies suggest different metrics depending on the use case, but we’ll focus on one key metric for each category to simplify our evaluation process.
Performance Metric
In AI, the Area Under the Curve (AUC) is a well-known concept. AUC provides a comprehensive measure of a model’s performance. It ranges from 0 to 1, with 0 indicating a useless model and 1 indicating a perfect one. AUC is particularly useful when evaluating model performance.
Fairness Metric
For fairness, the Disparate Impact (DI) metric is a common choice. DI measures the ratio between the probabilities of a positive outcome for unprivileged and privileged groups. Formally, DI is defined as:
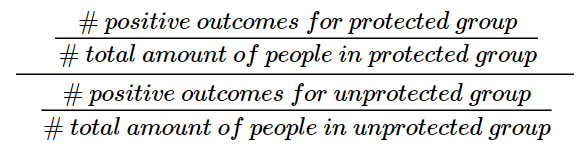
An ideal DI value is 1, indicating that both groups have an equal probability of a positive outcome. A value less than 1 suggests potential bias against the unprivileged group, while a value greater than 1 indicates potential bias in favour of the unprivileged group. This metric aligns with our definition of fairness as equality of opportunity, making it our standard measure of fairness for this blog series.
By using AUC to measure performance and DI to measure fairness, we can effectively evaluate and improve our machine learning models, ensuring they are both accurate and equitable.
The Concepts Are Few, And Methods Many
There are various classifications of biases, and accordingly, there are different methods to address them. These methods can be classified based on the phases of the machine learning pipeline: data preparation, training, and evaluation. This classification leads to three main strategies for tackling bias:
Pre-Processing Approaches
Pre-processing techniques modify the dataset before it’s fed into the model, aiming to remove or reduce biases at the data level. By ensuring the training data is as unbiased as possible, the models derived from it are less likely to propagate these biases. Methods might include re-sampling, re-weighting, or generating synthetic samples to create a balanced dataset.
In-Processing Approaches
In-processing methods adjust model parameters or the loss function during model training to minimize bias. These approaches work directly with the learning algorithm, integrating fairness constraints during the learning process to reduce the likelihood of biased outcomes.
Post-Processing Approaches
Post-processing techniques modify the model’s outputs after training to achieve fairness. These methods adjust model predictions based on fairness criteria, ensuring that final decisions align more closely with fairness objectives without needing to retrain the model.
In the following sections, we will explore a specific pre-processing approach to minimize AI bias.
Underrepresented groups == mistreated groups
Representation bias occurs when there is a disparity in the distribution of data among different subgroups within a dataset. A well-known example is the UCI Adult dataset, which exhibits biases related to the sensitive attributes of gender and race.
In the UCI Adult dataset, outcomes are categorized as high or low income (Positive and Negative). The dataset can be divided into eight distinct subgroups, each representing a unique combination of gender, race, and income outcomes. These subgroups are:
Gender:
- Male [M]
- Female [F]
Race:
- White [W]
- Non-White [NW]
Outcome:
- Positive [P]
- Negative [N]
Combinations:
- Negative Outcome White Males (N-M-W)
- …
- Positive Outcome Non-White Females (P-F-NW)
When examining the ratios of data points for each subgroup relative to the total dataset, it becomes clear that there is a significant imbalance. For example, the majority subgroup, Negative Outcome White Males, contains 14,608 data points, while the minority subgroup, Positive Outcome Non-White Females, contains only 168 data points out of a total of 36,178. This imbalance results in a significant disparity between privileged and unprivileged groups, reflected in average fairness during testing.
This raises the question of whether the observed bias is due to the imbalance in data distribution among subgroups. Currently, the distribution of data between subgroups is highly unequal. However, rebalancing the dataset so that each subgroup contains an equal number of data points could potentially mitigate this bias. For example, ensuring each subgroup has the same number of data points would involve increasing the representation of underrepresented groups and reducing the overrepresentation of majority groups.
In the following sections, we will explore a specific method to rebalance data distribution and its impact on reducing bias in AI systems and bias in ai models.
The Solution - Synthetic Data: A New Contender
Over the years, the challenge of dataset imbalances has spurred numerous solutions. While some suggest resampling subsets or under-sampling the majority group, these methods might compromise data integrity and model performance. Thus, the quest for a balance between fairness and performance continues. Fortunately, recent technological advancements have opened doors to innovative solutions.
The surge in data generation and synthesis methods offers an exciting avenue for dataset balancing. Instead of removing data for fairness, we can now generate additional data, minimizing bias while maintaining accuracy. Below is a schematic representation of our proposed bias-minimization methodology.
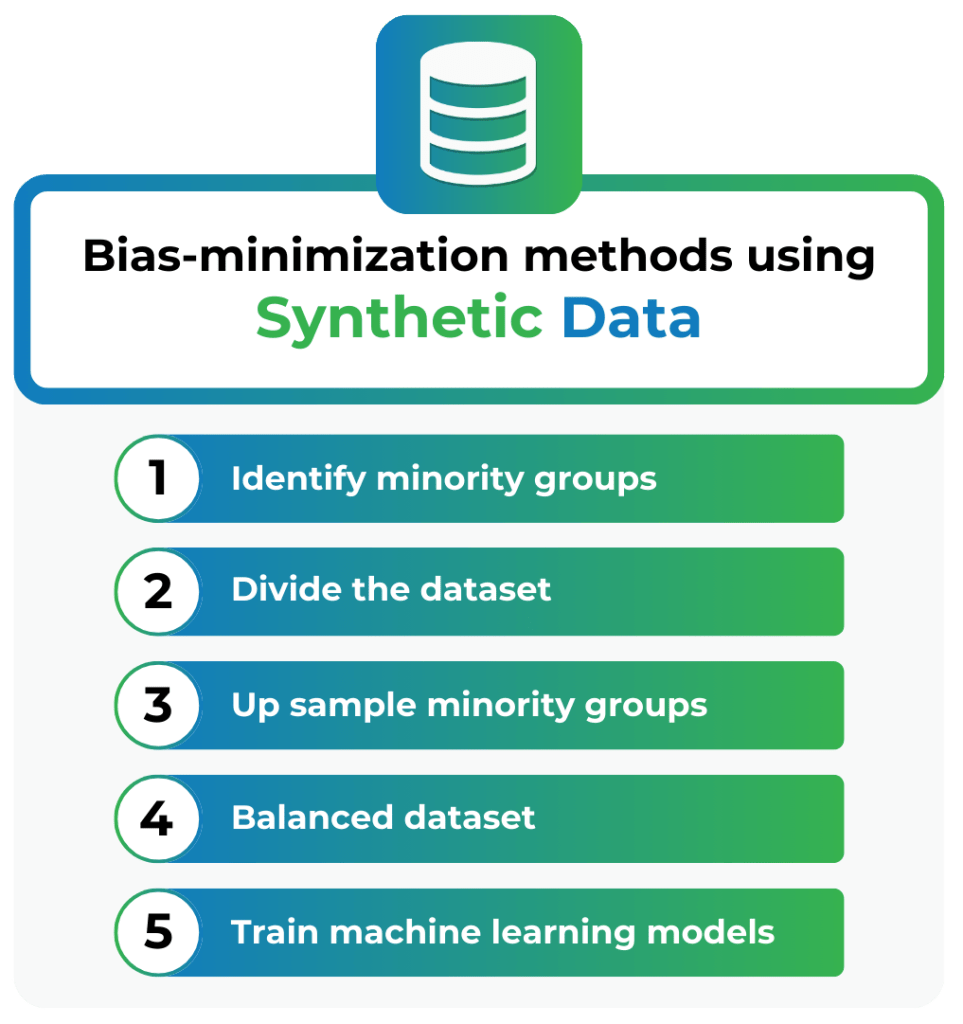
Bias-Minimization Methodology Using Synthetic Data
- Identify Minority Groups:
Analyze the dataset to identify minority groups based on sensitive attributes (e.g., gender, race, age). These sensitive attributes are columns in the dataset that may induce bias.
- Divide the Dataset:
Divide the dataset into n different subgroups, each representing a unique combination of sensitive attributes.
- Up sample Minority Groups:
Identify the minority group(s) within these subgroups and generate synthetic data to up-sample the minority groups until they have the same number of data points as the majority group.
- Balanced Dataset:
Combine the synthetic data with the original data to create a balanced dataset.
- Train Machine Learning Models:
Use the balanced dataset to train machine learning models, following standard training procedures.
The Testing Pipeline
While we have discussed specific performance and fairness metrics, it is essential to emphasize that no single metric perfectly encapsulates bias. Each metric provides a unique perspective. For instance, the Equal Opportunity Difference (EOD) offers insights into the difference in True Positive Rates (TPR) for privileged and unprivileged groups, while Disparate Impact (DI) focuses on the ratio of favourable outcomes between these groups. Their subtle differences make them collectively crucial for painting a comprehensive picture of bias.
These nuances necessitate a robust testing pipeline that evaluates both performance and fairness. Below is an outline of this comprehensive framework.
Elaborating on the Testing Pipeline
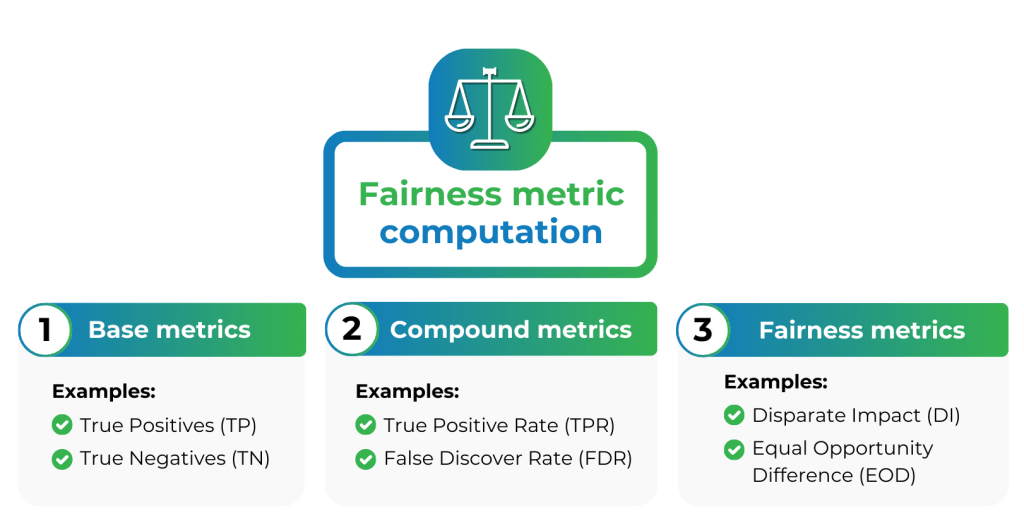
Fairness metric computation can be broken down into three distinct groups:
- Base metrics such as True Positives (TP), True Negatives (TN) etc.
- Compound metrics such as True Positive Rate (TPR), False Discover Rate (FDR), etc.
- Fairness metrics such as Disparate Impact (DI), Equal Opportunity Difference (EOD), etc.
Given our focus on evaluating privileged and unprivileged groups separately, it’s essential to compute their metrics distinctively. For this, we iterate over all sensitive attributes and separate the test data points for each group. This allows us to calculate confusion matrices for each test dataset subset individually. We store these results in a dictionary for easy reference.
Once we’ve derived these base metrics, we repeat a similar process for the compound metrics. After calculating these for each sensitive attribute subgroup, we integrate them into fairness metrics. The results we present later are averages of fairness metrics across different sensitive attributes, offering a concise understanding of the model’s efficacy. Additionally, we also evaluate the performance of the model (with the AUC) with the test data as a whole.
The Results
Tests using our proposed framework reveal significant efficacy in bias minimization. In some scenarios, we observed up to 2x improvements in the fairness metric DI. In other metrics, the gains were as high as tenfold, all while preserving the AUC score and incurring minimal accuracy loss. Some of these findings are visualized below.
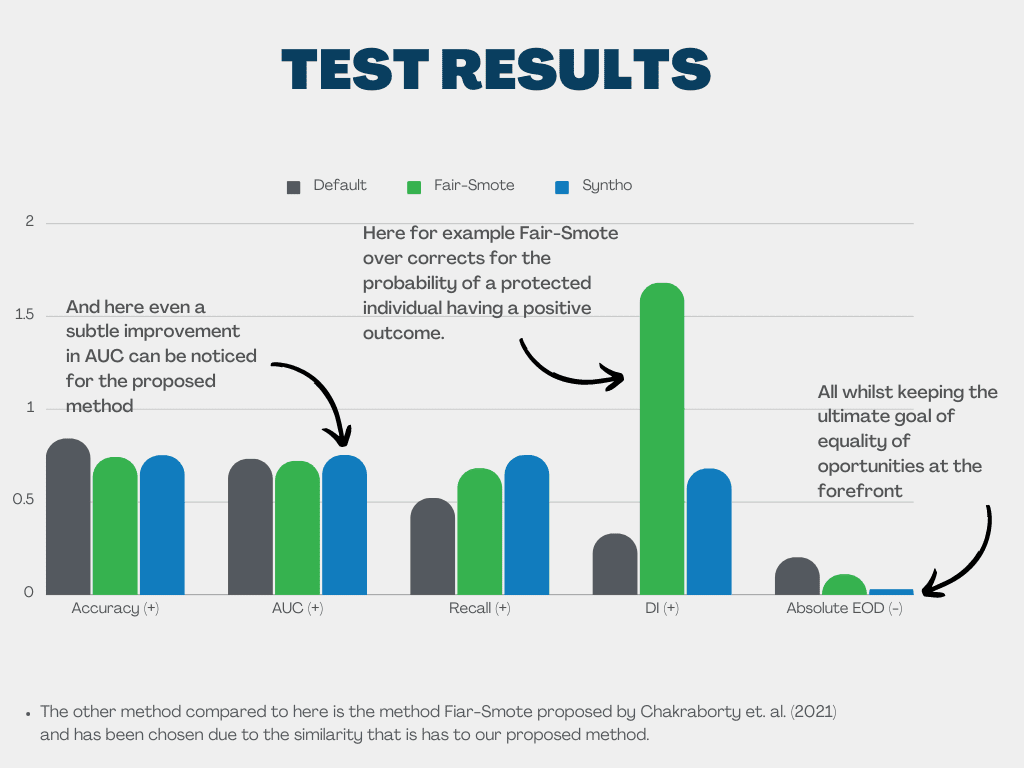
However, there are crucial insights to consider:
- Model specificity: As we’ve discussed, biases can emerge from model selection. Our tests revealed models like Random Forest and Gradient Boosting performing best with default hyperparameters. However, model fine-tuning remains a promising area for further exploration.
- Sensitive attributes matter: During testing, specific combinations of sensitive attributes resulted in significant accuracy losses with negligible fairness improvements. This emphasizes the need for careful attribute selection.
- Model efficacy depends on data and use-case: While our model has demonstrated efficacy across various scenarios, its effectiveness is contingent on the quality and nature of the input data. For instance, if a dataset inherently lacks representation from certain subgroups, bias minimization for those groups becomes infeasible. This reinforces the idea that every step in the machine learning pipeline should be crafted to minimize biases.
Summary
Tests using our proposed framework reveal significant efficacy in bias minimization. In some scenarios, we observed up to 2x improvements in the fairness metric DI. In other metrics, the gains were as high as tenfold, all while preserving the AUC score and incurring minimal accuracy loss. Some of these findings are visualized below.
As AI continues to evolve, the focus on eliminating bias must intensify. This involves continuous research, the development of more sophisticated techniques, and rigorous regulatory frameworks. We live in an inherently biased world, and as Hellström et al. succinctly posed: do we want to model the world as it is, or as we would like it to be? Evaluating on biased data will favour biased models, making it crucial to stay updated with new evaluation metrics.
Our proposed framework is not the “golden standard,” but a significant step toward minimizing biases in machine learning models. This process is iterative, and no single solution will work for everyone. The key is to keep searching for ways to build a more fair future together.
About Syntho
Syntho provides a smart synthetic data generation platform, leveraging multiple synthetic data forms and generation methods, empowering organizations to intelligently transform data into a competitive edge. Our AI-generated synthetic data mimics statistical patterns of original data, ensuring accuracy, privacy, and speed, as assessed by external experts like SAS. With smart de-identification features and consistent mapping, sensitive information is protected while preserving referential integrity. Our platform enables the creation, management, and control of test data for non-production environments, utilizing rule-based synthetic data generation methods for targeted scenarios. Additionally, users can generate synthetic data programmatically and obtain realistic test data to develop comprehensive testing and development scenarios with ease.
Syntho wins Unesco’s Gender Bias Challenge at Vivatech 2021 in France, read more.
Do you want to learn more practical applications of synthetic data and how to fight bias ai? Feel free to schedule a demo!
About the authors
Software Engineering Intern
Roham is a bachelor student at the Delft University of Technology and is a Software Engineering Intern at Syntho.
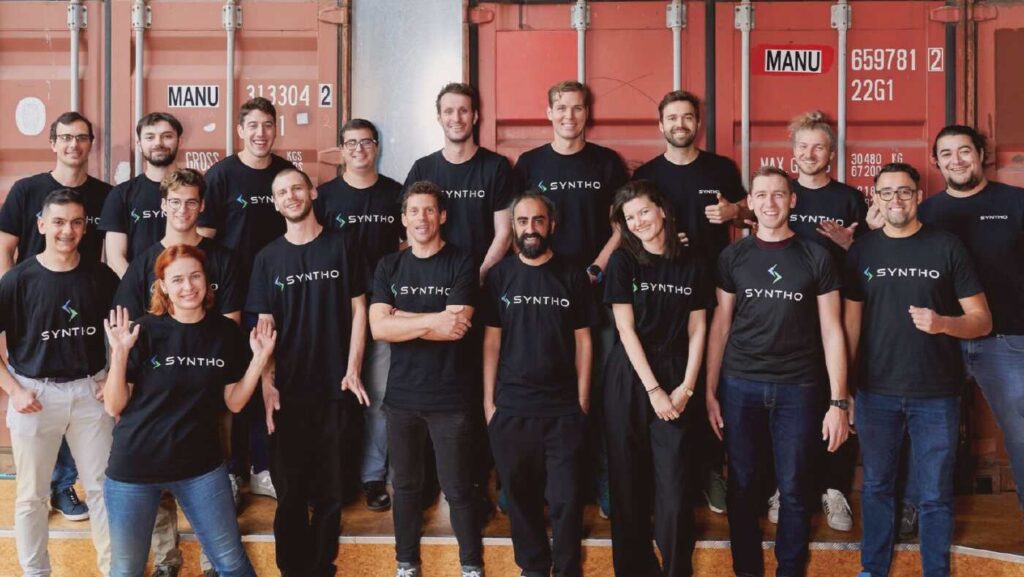
Data is synthetic, but our team is real!
Contact Syntho and one of our experts will get in touch with you at the speed of light to explore the value of synthetic data!